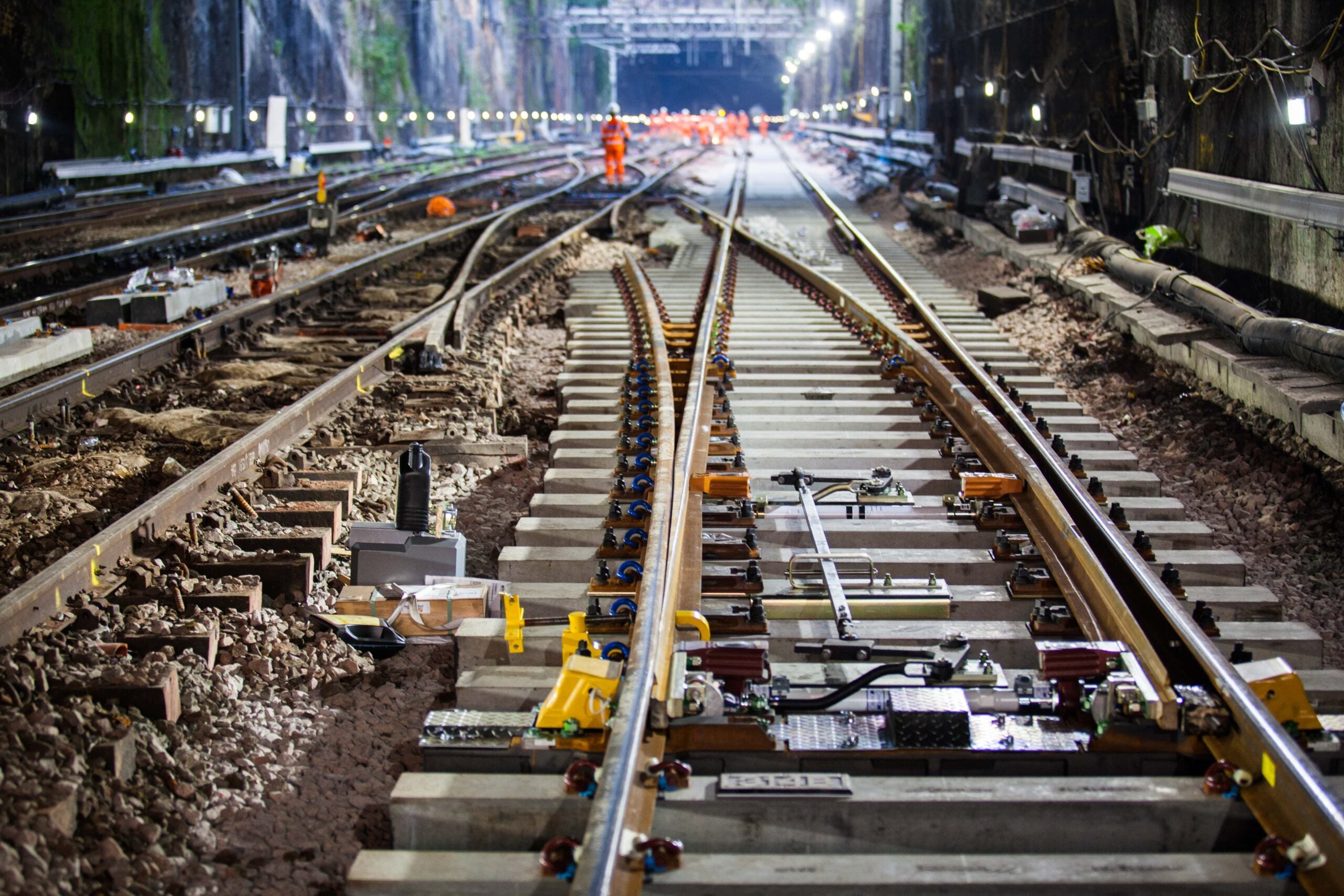
Smart maintenance systems that are currently in use by the railway industry allow the detection and discovery of faults on the line via the capturing of asset knowledge through point machines. An alarm is triggered if levels reach a particular threshold level, alerting staff of the fault, leaving them to work out the most likely cause. However, this technology is not always the most accurate, with staff receiving false alerts and, conversely, faults being missed.
Thales, a French multinational company that designs and builds electrical systems and services for a range of industries, has developed a new smart maintenance system for rail and network operators.
Thales Transport UK product line manager, Nathan Marlor, explains the benefits of the new technology, and how the system works.

Frankie Youd (FY): What is Thales ‘Points Predict’ and what does it aim to achieve?
Nathan Marlor (NM): Five or six years ago, there was a consensus that monitoring the condition of point machines was beneficial. It allowed those in the industry to have insight into the behaviour of the point machine. They could look at the data, they could then determine whether the point machine was operating normally or abnormally and take some action based on that.
How well do you really know your competitors?
Access the most comprehensive Company Profiles on the market, powered by GlobalData. Save hours of research. Gain competitive edge.
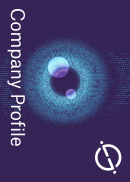
Thank you!
Your download email will arrive shortly
Not ready to buy yet? Download a free sample
We are confident about the unique quality of our Company Profiles. However, we want you to make the most beneficial decision for your business, so we offer a free sample that you can download by submitting the below form
By GlobalDataBut all the systems that are out there today from all the different providers work on a concept of threshold-based notifications. If a certain parameter goes over a certain threshold you get a notification or alarm. A typical example would be if the point machine used more than five amps of average current, you might set your threshold to say six and then you will get a notification or an alarm, based off that.
They’ve got more and more complex which is great because you can get more and more accuracy, but with that comes a lot of management.
What we now see across the industry is not just one or two parameters it can be up to ten for each individual point machine. In the UK we know that Network Rail is monitoring around 14,000 point machines so that suddenly becomes quite a big job.
That was our challenge as a team; our data science team went away and started to work on this Points Predict algorithm. What our algorithm aims to do is remove any of that complexity of having to set thresholds or change them or continually tweak them depending on the variability, it does it all automatically. There is now just one single parameter to tweak across the whole network, and that’s it.
FY: How does the technology work?
NM: We offer Points Predict in two different ways. If the customer doesn’t have any condition monitoring systems, we can provide a turnkey solution through our software model, that gets them up and running almost immediately.
If a system already exists, we’ve proven that it’s possible to integrate into that existing system and apply this new algorithm to the existing data. It doesn’t mean that we have to rip out all the existing sensors and hardware we can kind of just bolt it on top.
In terms of the algorithm itself we use artificial intelligence, we use clustering, unsupervised machine learning – effectively there’s two algorithms at play within Points Predict. There is an unsupervised machine learning algorithm, which doesn’t need any training, so you don’t need to provide it with hundreds of examples of faults. It kind of buckets the data, that’s the best way that I like to imagine it, it buckets some data into the normal bucket and some data into a fault bucket and carries on like that.
The second algorithm is a supervised algorithm. Effectively, what the system does when it buckets something outside of that normal it asks the operator what type of fault that is, so it just asks once and then from there it’s got a 90% accuracy on the recall of it.
FY: What are the main benefits of this new system over the previous technology?
NM: What I asked our data scientists to do is use a big set of data that we had access to and do like for like comparison between if you use the current threshold technique versus the algorithm, what benefits would we see.
They came back and said it is a 50% improvement in detection rate, so if you were using the old threshold-based system you would effectively have lost 50% of the anomalies, you would have missed them.
We found a 75% reduction in false alerts, point machines are subject to quite a lot of variability. If it’s the first time they’ve been operated in the day we know that they use a lot more power than the second or third time because they warm up a bit – and they use a lot more power over winter. This reduction rate is a huge benefit for the operators, they don’t need to wade through the data and work out what the genuine alerts are, and which ones aren’t.
As I mentioned it has 90% classification accuracy, we don’t need expert sat in front of the system, applying their knowledge in the context permanently anymore. We can ask them to look at a piece of data once, train the system and then from there it will carry on doing the job for us effectively.
FY: What steps were taken to design the new system?
NM: When we talk about the platform in general, we tend to talk about five key benefits, I think Points Predict supports every one of those. We talk about early awareness, so being able to give our operators a bit of a heads up that something’s going wrong.
We talk about right fix first time, understanding the problem before you get there so you can make sure you go with the right spares or the team with the right qualifications. The last thing you want to do is turn up to the site and not understand what the fault is and maybe miss something.
We talk about protecting staff so if we can reduce the amount of time that teams have spent trackside, that’s a huge safety improvement. This one’s been a real key for us and our customers through the lockdowns as well. As it’s a cloud-native application the operators can get the same data at their home office, on their phone or in a coffee shop, as they could if they were stood by the asset trackside or within the equipment room.
Finally, real-time assistance. We talk about the fact that now we can share data with experts within the supply chain in this real-time scenario where if we’ve got a complex problem we’re not having to send data over email screenshots anymore, everyone can log on to the platform and look at the same data and come up with some kind of collaborative diagnosis.
FY: Is training required or can operators use this technology straight away?
NM: We’ve tried to make it as intuitive as possible. A lot of the way that the teamwork is centred around this design thinking approach which brings the end-users in right from the very start. This ensures that we’ve got an intuitive, easy to use user interface, so they don’t need weeks and weeks of training.
It’s the same as if you open any kind of app on your Facebook or your Twitter you kind of know where the buttons are, and you know what they do. That’s not by chance that’s through lots of user research and testing, and we’ve done the same thing with our Points Predict user interface to make sure that it’s as simple as possible for those using it to interpret the results of the algorithm and then act.